ADVANCES
Assessments of natural capital (e.g. assets such as landscapes, soils, water, air and ecosystems) are now widely incorporated into public and private sector decision-making to allow comparison of potential policies or projects in terms of changes in the benefits (e.g. regarding food supply, water purification, flood protection, carbon storage, and recreation and amenity experiences) provided to society.
However, application of this approach faces several challenges, such as those related to scale effects, trade-offs between benefits and uncertainties in model estimates.
Principle Investigator Andrew Lovett introduces the ADVANCES project in this video
ADVANCES involves a team of natural capital researchers and applied mathematicians or statisticians from seven institutions. It seeks to build collaborations between these disciplines to develop better computer-based optimisation and statistical tools to assess changes in natural capital. The objectives of the project are to:
- Incorporate decision making under uncertainty into spatial optimisation tools for natural capital decision making. This will focus on food production and biodiversity trade-offs under different pathways of climate change and agricultural prices.
- Identify strategies to address modelling issues such as context dependency, cumulative effects and uncertainty propagation in the context of managing landscapes for multiple benefits related to natural capital. This will include a focus on large scale afforestation scenarios in Britain.
- Implement new methods for the operational assessment of ‘hard-to-value’ landscape assets, specifically biodiversity and cultural ecosystem services.
- Engage with prospective users of more advanced modelling tools in government departments, businesses and other organisations concerned with land management and nature conservation in order to help ensure that the findings and modelling techniques produced by ADVANCES are relevant to their needs and ultimately better future decision-making about the UK Landscape.
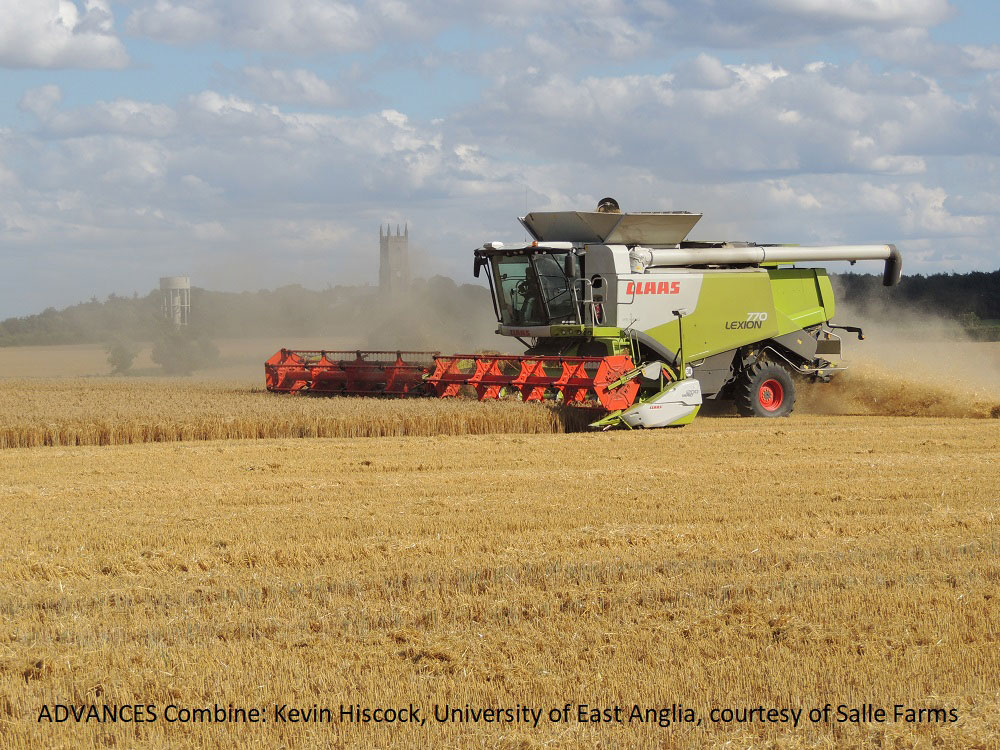
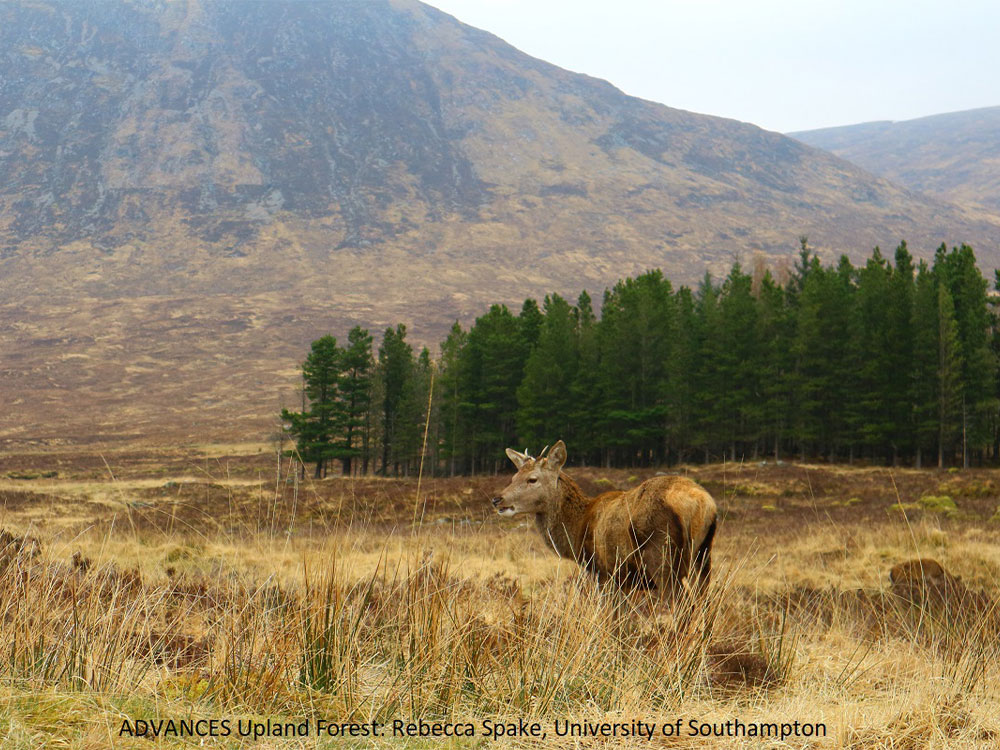
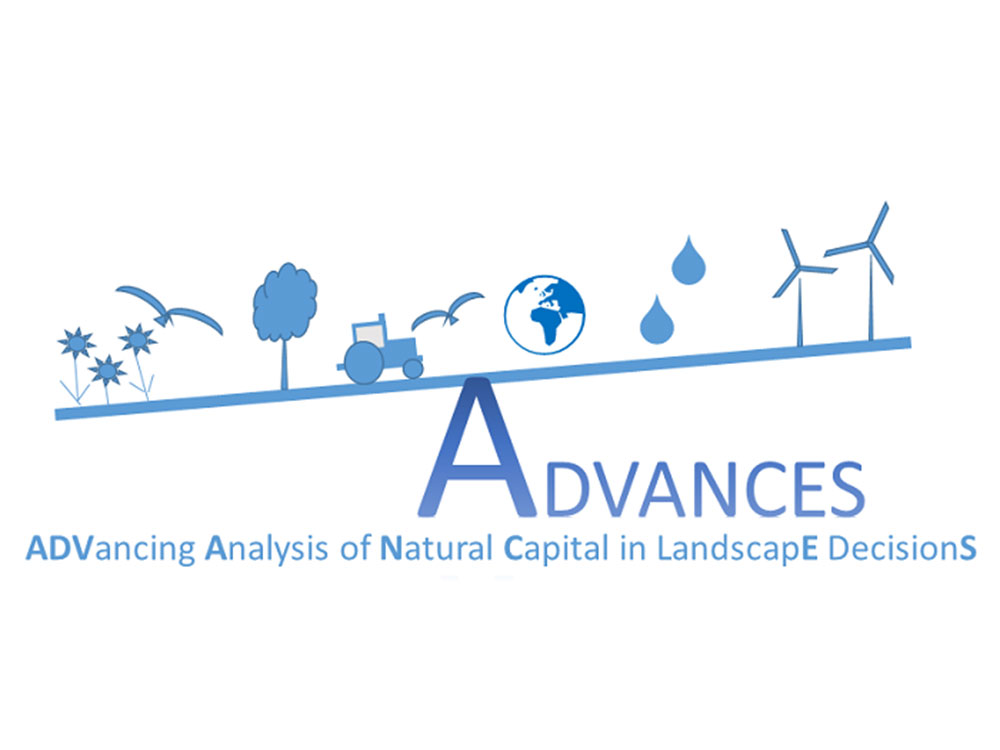