EnsemblES
Using ensemble techniques to capture the accuracy and sensitivity of ecosystem service models
Nature is vital for the wellbeing of people. We depend on nature for food, water, medicine, and recreation. We call these contributions ‘ecosystem services’.
To make sustainable decisions, we need to know how these services are affected by our actions. However, despite their importance, the information available to measure ecosystem services remains limited.
Using recent advances in data availability, new models are increasingly able to provide credible information where empirical data are lacking. These models can produce maps of estimated ecosystem services which can then be used by decision-makers.
However, most attempts to map ecosystem services in this way rely on a single model, and few applications validate these models against independent datasets. As a consequence, the uncertainties associated with applying these models remain largely unknown. This is important because the results of models that are validated at the local level are unlikely to be transferable to other locations or to the regional and national level, where the outputs of models are most widely used.
Project aims
The aim of the EnsemblES project was to address these issues by assessing whether combining the outputs from multiple models into ‘ensembles’ of models can provide more accurate predictions than individual models alone. The project also sought to identify which methods of combining models into an ensemble are most applicable for the study of ecosystem services; highlighting the sensitivity of models (and ensembles of models) to input data. Finally, it evaluated the accuracy of these methods in data-deficient areas. EnsembIES project research fed into studies published in Ecosystem Services, Ecosystems, Nature Communications, One Earth, and Science of The Total Environment, as well as datasets for the UK and sub-Saharan Africa published by the NERC Environmental Information Data Centre.
Conclusions
The project found that ensembles of ecosystem service models can indeed improve accuracy and indicate uncertainty. Researchers used validation data across a data-deficient area to test the accuracy of ensembles of models against the accuracy of individual models. This comparison found evidence that had at minimum a 5–17% higher accuracy than a randomly selected individual model and, in general, ensembles weighted for among model consensus provided better predictions than unweighted ensembles.
Although sometimes individual models can give good predictions, without validation against data or other models there is the potential for serious negative consequences to occur if estimates deviate significantly from the truth. For this reason, ensembles of models should be widely adopted so that sustainable development decisions can be made to protect the ecosystem services we all rely on. Our analysis suggests various ensemble methods should be applied depending on data quality, for example if validation data are available.
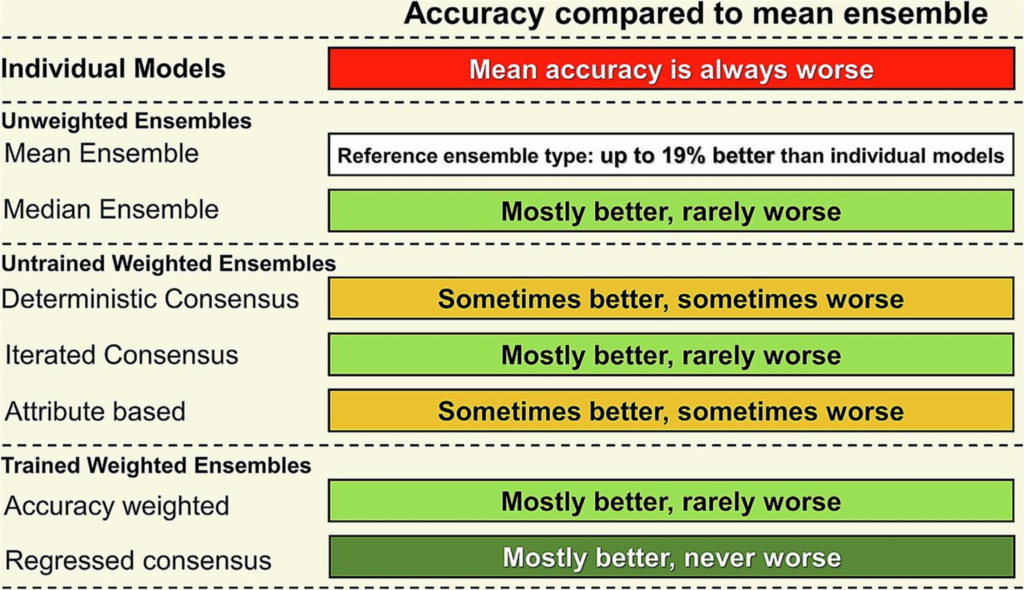
Project outputs
- ‘Model ensembles of ecosystem services fill global certainty and capacity gaps‘ in Science Advances, Volume 9(14), April 2023.
- ‘Reducing uncertainty in ecosystem service modelling through weighted ensembles’ in Ecosystem Services, Volume 53, February 2022, 101398.
- ‘Ensemble outputs from Ecosystem Service models for water supply, aboveground carbon storage and use of water, grazing, charcoal and firewood by beneficiaries in sub-Saharan Africa’ via NERC Environmental Information Data Centre, 25 June 2020. [Project dataset available under the terms of the Open Government Licence].
- ‘Ensembles of ecosystem service models can improve accuracy and indicate uncertainty’ in Science of The Total Environment, Volume 747, 10 December 2020, 141006.
- ‘Nature provides valuable sanitation services’ in One Earth, Volume 4(2), 19 February 2021.
- ‘A Continental-Scale Validation of Ecosystem Service Models’ in Ecosystems, Volume 22, 22 April 2019.
- ‘Regime shifts occur disproportionately faster in larger ecosystems’ in Nature Communications, Volume 11, 10 March 2020.