Explainable AI for UK agricultural land use decision-making
Spatial land use models are indispensable for providing scientific evidence that can inform sustainable land use planning.
However, understanding and predicting agricultural land use change and the factors that drive such change is difficult due to the highly complex systems of flows that are subject to non-linearities, sensitivities, and uncertainties across spatial and temporal scales. This complexity is challenging to represent using traditional statistical modelling approaches.
Principal Investigator Paula Harrison introduces the project in this short video
This project explored and tested the ability of Artificial Intelligence (AI) techniques to complement traditional land use modelling approaches by learning complex spatial and temporal relationships between large geospatial land use datasets and datasets of important driving factors of land use change.
The project was an exploratory study that aimed to demonstrate proof-of-concept for selected regions in the UK and provide greater understanding of the state and dynamics of agricultural land use systems and how they can be influenced by policy and management decisions.
Key findings
The project developed a novel approach that combines the AI deep learning method, known as Generative Adversarial Networks, with a traditional land use modelling approach, known as Cellular Automata models. The project team tested the improvement in predicting land use changes from 1990 to 2019 using UKCEH Land Cover Map data to assess if the inclusion of the AI method provided better characterisation of spatial patterns and landscape dynamics. They investigated the method using three categories of driving factors, which are thought to be particularly important determinants of UK land use change: climatic factors (temperature, precipitation, evapotranspiration), topographic factors (elevation, slope, distance to water courses) and human-related factors (distance to urban areas, distance to major roads, distance to railways).
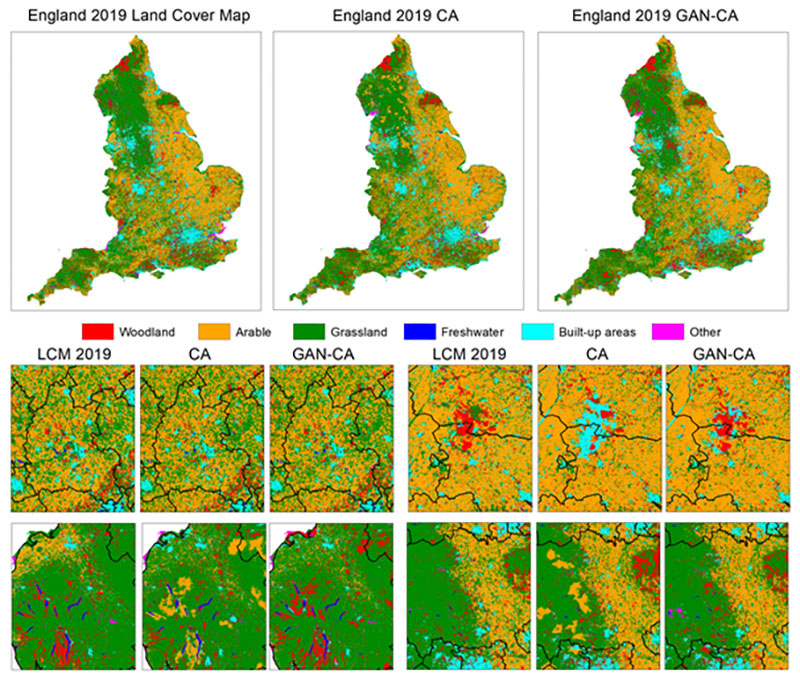
The figure shows the original UKCEH Land Cover Map data for 2019 for England (LCM 2019) and predicted land cover for 2019 based on 1990 data and the three categories of driving factors for the Celluar Automata (CA) model alone, and for the new approach combining Generative Adversarial Networks with the CA model (GAN-CA)
The key findings identified in the project included first, that the Cellular Automata model was able to predict future land use change. However, it only utilised limited neighbourhood information at fine scales without considering the large-scale heterogeneous environment. The transition between arable and grassland land cover classes was particularly challenging to predict using a Cellular Automata model, with relatively low accuracy (71%).
Second, the project found evidence that capturing fine resolution spatial patterns and their context are essential for predicting land use changes. This was demonstrated in both a pilot study in Oxfordshire and wider application of the project’s method for England. The project team demonstrated that in both case studies accuracy of predicting land use change was increased by 8% with the incorporation of spatial and context information.
Third, combining the AI (deep learning) method with the Cellular Automata model provided both fine-scale neighbourhood and large-scale context information to make joint landscape decisions. The spatial patterns among woodland, arable, grassland, freshwater and urban are accurately characterised, with an overall accuracy of 89%.
Finally, human-related factors were found to be key drivers of land use changes. In particular, the distance to urban and distance to road were the two most important drivers that have significant impact on land use changes. These drivers are highly related to human activities and road accessibility, and influenced UK landscape dynamics over the past 30 years.