Issues of Uncertainty and Scale in Derived Products
Landscape decision-makers and modellers require data to understand how natural and anthropogenic properties vary in space. They also require data to decide which areas are suitable for specific infrastructure and activities or where hazards might occur.
Organisations including the British Geological Survey provide such data as gridded products that map factors such as topography, land use, soil properties and weather variables. These products are normally provided at a single or small number of scales or resolutions and do not include information about uncertainty.
Principal Investigator Ben Marchant introduces the project in this short video
Different users of the products require information at different spatial scales. For example, decision-makers concerned about the risk of a landslide occurring on a particular hillside might only be interested in rainfall data for the immediate locality. In contrast, water managers interested in the quantity of groundwater within an aquifer might utilise rainfall data from across a wider catchment. The uncertainty in data products propagate through data processing and modelling procedures leading to unreliable decisions.
The products are often derived from a sample of measurements of the property of interest which are interpolated to cover the entire country or region of interest. The rainfall maps in Figure 1 are derived from UK Met Office (2019) rain gauges. If this interpolation is performed using geostatistical methods then it is possible to determine the resultant uncertainty of the derived maps (Figure 2).
Figure 1: Interpolated rainfall maps derived from Met Office (2019) data (provided under licence)
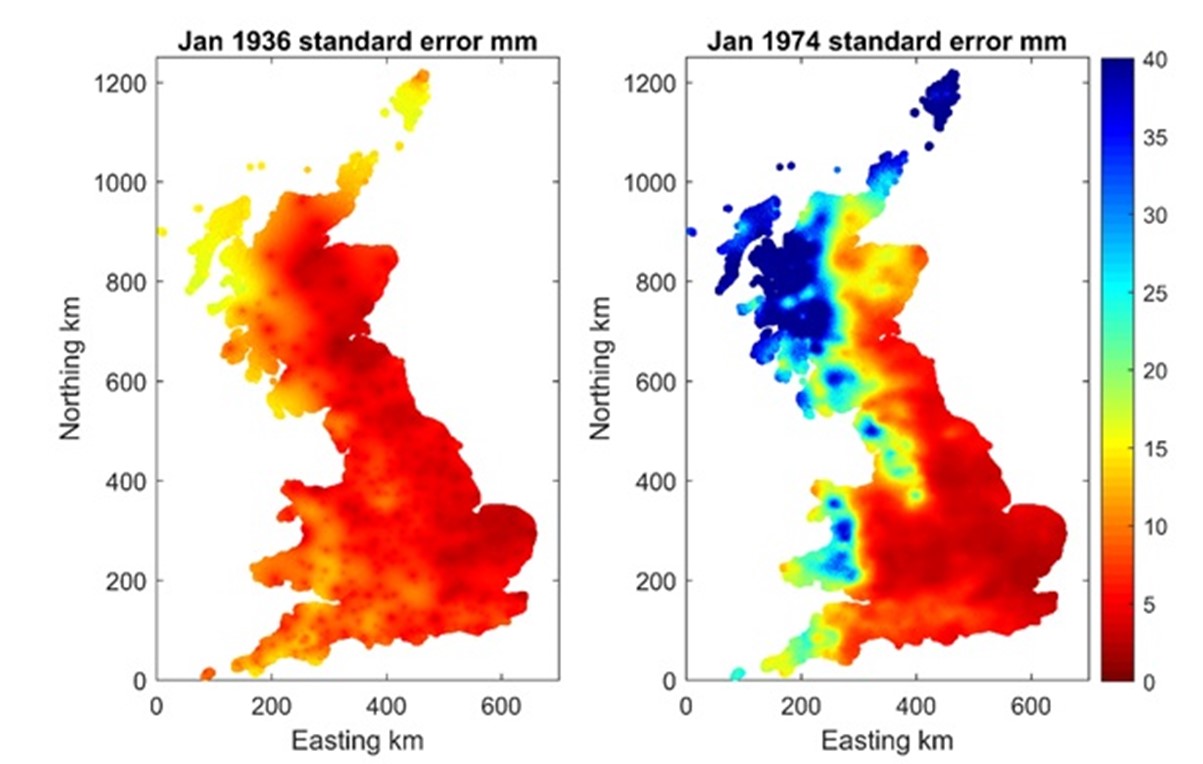
Figure 2: Spatially varying uncertainty of each rainfall map
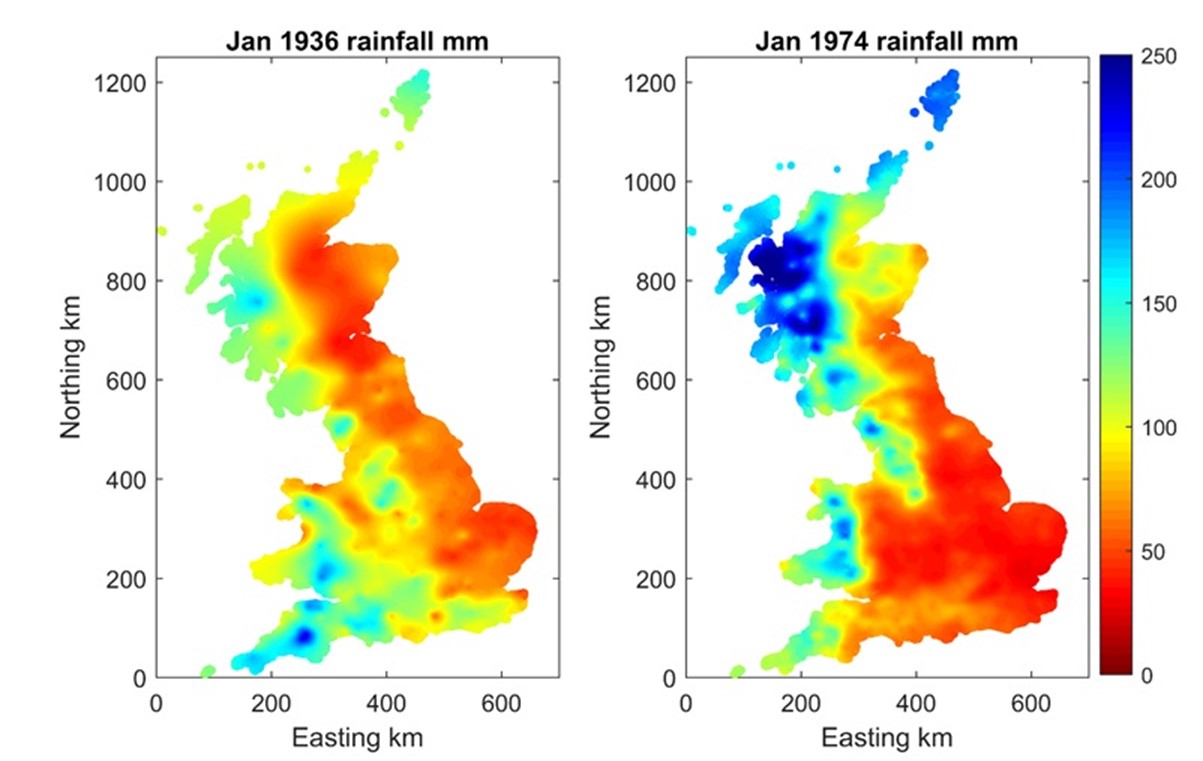
However, this uncertainty computation can be time-consuming (particularly for products that vary in both time and space) and the uncertainty varies according to the scale of the required output, as shown in Figure 3 below.
Figure 3: The relationship between spatial scale of the output map and the uncertainty (width of the confidence level) for the site of a groundwater measurement borehole
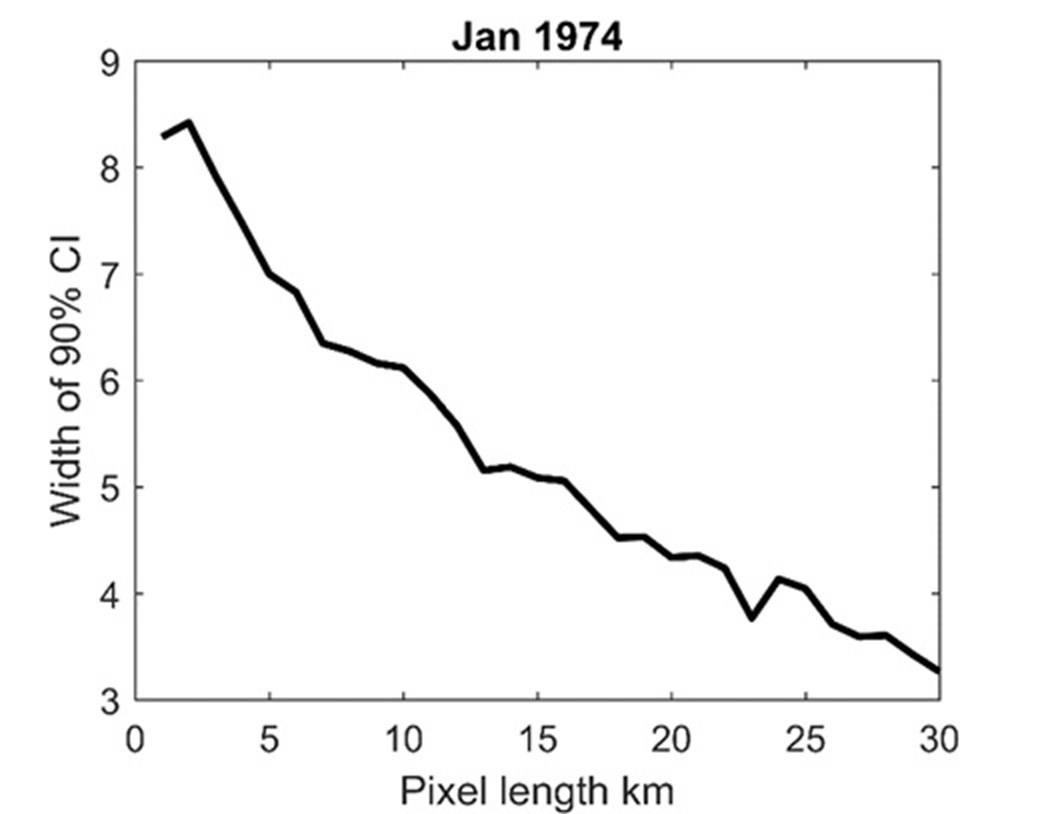
This project was concerned with providing information relevant to decision-makers across all scales and in quantifying the uncertainty in this information. Statisticians explored computationally efficient methodologies to produce multi-scale products, while data scientists and software developers suggested approaches by which these can be distributed. The propagation of uncertainty in data products through process models of groundwater levels was explored and quantified. The number and arrangement of measurements required to produce multi-scale products was also determined.
Key contributions
The implications of uncertainty were explored in the context of peat quality mapping. Surveys of peat depth were used to demonstrate how the uncertainty in mapped products varies according to the spatial-scale or resolution at which information is required. New statistical approaches were developed to quantify this multi-scale uncertainty and to explore how uncertainty in one mapped product propagates into other related products. Novel methods for designing environmental surveys were developed based on these statistical approaches to minimise the uncertainty in the resultant mapped products. This led to an approach to survey peat depth that is considerably more cost-effective than current standard practice.
The approaches for efficient mapping of peat depth developed in the project were circulated amongst the DEFRA steering committee overseeing the production of a UK peat map and BGS and Natural England have discussed how these approaches should be adopted within this endeavour. More generally, the statistical methodology developed in the project can be used to assess the uncertainty of any derived map of an environmental property, to identify where more data is required to improve this map and design the required data survey. This will improve the accuracy of all subsequent modelling and land assessment exercises that use these datasets.
Project contacts
B P Marchant
(Principal Investigator)
Russell Lawley
(Co-Investigator)
Andrew Finlayson
(Co-Investigator)
Project outputs
- Using remote sensors to predict soil properties: Radiometry and peat depth in Dartmoor, UK, Geoderma, December 2021.
- Estimating organic surface horizon depth for peat and peaty soils across a Scottish upland catchment using linear mixed models with topographic and geological covariates, Soil Use and Management, April 2020.