Quantifying uncertainty in the predictions of complex process-based models
Decisions about land use require that trade-offs between competing demands be identified, and their consequences through time be characterised. Often this is done with reference to maps.
Methods for representing consequences through time on maps may require complex models such as stochastic computer simulations. Such models are increasingly used to make realistic predictions about real world processes. Because these models attempt to simulate all relevant aspects of a real physical system, they may involve many parameters, some of which will be difficult to set correctly. As the final objective of these models is to assess the possible consequences of management decisions, such as the placement of roads, wind turbines or flood defences, it is crucially important that the uncertainty introduced by calibrating parameters be understood.
Co-Investigator Richard Everitt introduces the project in this short video
Approximate Bayesian Computation, or ABC, is a promising technique for estimating parameter values together with their credible intervals, and this allows calculation of the uncertainty deriving from parameter calibration. This project developed improved ABC methods to make them fast and accurate enough to be widely used, both to calibrate and evaluate complex stochastic computer models, and to quantify the uncertainty attached to their predictions.
The project was able to fit and evaluate the accuracy of complex models for real, challenging applications, and has laid the groundwork for this approach to be more widely used in practice. Members of the project team contributed to a publication in Ecological Modelling that uses the approach to model European sea bass.
The project team are keen that their methods be useful to all those making decisions about landscapes. If you have a model that would benefit from uncertainty quantification, please get in touch.
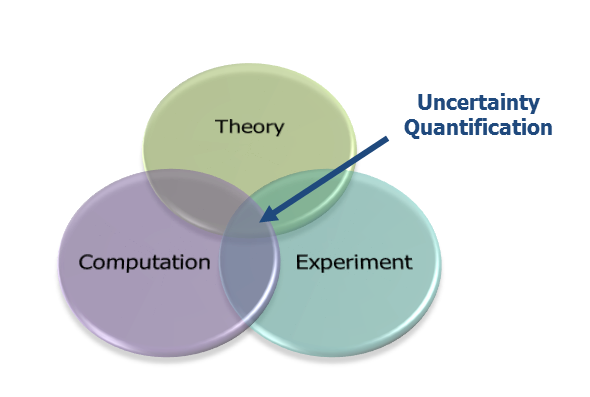
Project contacts
Prof Richard Sibly
(Principal Investigator)
Dr Richard Everitt
(Co-Principal Investigator)
Project outputs
- Incorporating environmental variability in a spatially-explicit individual-based model of European sea bass, Ecological Modelling, Vol. 466, April 2022.